How AI and Predictive Analytics Are Transforming Solar Energy Management
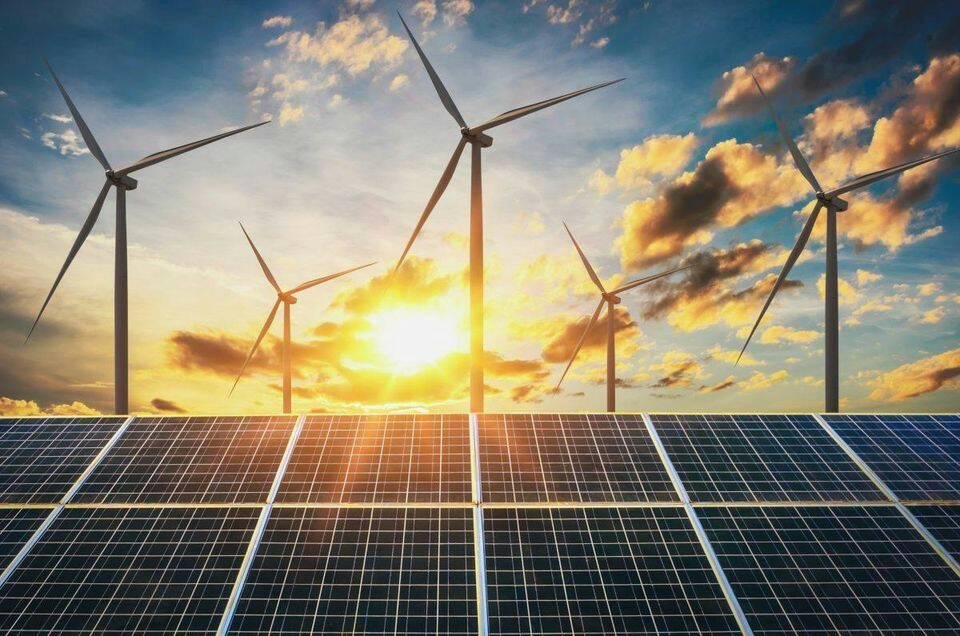
The solar energy industry has long been hailed as a beacon of sustainability, offering clean, renewable power to combat climate change and reduce reliance on fossil fuels. However, as the adoption of solar energy accelerates globally, managing its complexities—such as intermittency, grid integration, and operational efficiency—has become increasingly challenging. Enter artificial intelligence (AI) and predictive analytics: transformative technologies that are revolutionizing how we design, operate, and optimize solar energy systems. This article explores the pivotal role these innovations play in reshaping solar energy management and driving the transition to a smarter, more sustainable energy future.
1. The Growing Complexity of Solar Energy Systems
Solar energy systems have evolved from simple rooftop installations to sophisticated networks of distributed generation, utility-scale solar farms, and hybrid renewable energy systems. While this growth is promising, it introduces new challenges:
- Intermittency: Solar energy production depends on weather conditions, time of day, and seasonal variations.
- Grid Stability: Integrating fluctuating solar output into existing power grids requires advanced monitoring and control mechanisms.
- Maintenance Demands: Large-scale solar arrays require regular upkeep to ensure peak performance, which can be labor-intensive and costly.
To address these challenges, the solar industry is turning to AI and predictive analytics. These tools enable data-driven decision-making, automate processes, and enhance system performance, unlocking unprecedented levels of efficiency and reliability.
2. How AI Enhances Solar Energy Management
AI refers to machines or software capable of mimicking human intelligence to perform tasks such as learning, reasoning, and problem-solving. In the context of solar energy, AI applications span multiple domains:
a. Optimized Energy Production Forecasting
One of the most significant contributions of AI is its ability to predict solar energy generation with remarkable accuracy. Machine learning algorithms analyze vast datasets, including historical weather patterns, real-time meteorological data, satellite imagery, and local irradiance levels. By identifying trends and correlations, these models forecast solar output hours, days, or even weeks in advance.
Accurate forecasting enables utilities to better balance supply and demand, reducing reliance on backup fossil fuel generators and minimizing curtailment (the deliberate reduction of solar output when there’s excess supply). For example, Google’s DeepMind AI has demonstrated success in predicting wind power output up to 36 hours ahead—a similar approach is now being applied to solar farms.
b. Smart Grid Integration
As solar becomes a larger share of the energy mix, maintaining grid stability becomes crucial. AI-powered smart grids use predictive analytics to anticipate fluctuations in solar generation and adjust accordingly. For instance:
- Demand Response Optimization: AI algorithms analyze consumer behavior and dynamically shift non-critical loads to periods of high solar availability.
- Voltage Regulation: Advanced sensors and AI controllers stabilize voltage levels across the grid, preventing overloads during peak solar hours.
- Energy Trading Platforms: Blockchain-enabled platforms powered by AI facilitate peer-to-peer energy trading, allowing prosumers (consumers who also produce energy) to sell excess solar power directly to neighbors or utilities.
These innovations ensure seamless integration of solar energy while enhancing overall grid resilience.
c. Predictive Maintenance
Traditional maintenance schedules for solar panels often involve manual inspections or routine check-ups, which may miss emerging issues until they escalate. AI-driven predictive maintenance leverages IoT sensors embedded in solar panels and inverters to monitor performance metrics such as temperature, voltage, current, and shading effects.
By analyzing this data, AI identifies anomalies indicative of faults, degradation, or potential failures before they occur. For example:
- A sudden drop in panel efficiency might signal dirt accumulation or wiring issues.
- Elevated temperatures could indicate overheating components requiring immediate attention.
Predictive maintenance not only extends the lifespan of solar assets but also reduces downtime and repair costs, improving return on investment for operators.
3. Real-World Applications of AI in Solar Energy
Numerous organizations and startups are already leveraging AI to transform solar energy management:
a. Tesla Autobidder
Tesla’s Autobidder platform uses AI to optimize the dispatch of solar and battery storage systems in real-time. By predicting electricity prices and grid demand, the system maximizes revenue for solar farm owners while stabilizing the grid.
b. Nextracker TrueCapture
Nextracker’s TrueCapture technology employs AI to optimize the positioning of solar trackers throughout the day. Using machine learning, the system accounts for factors like cloud cover, terrain shadows, and diffuse light to maximize energy yield. Customers report gains of up to 6% in annual energy production.
c. IBM’s Hybrid Renewable Energy Forecasts
IBM Research developed an AI-powered tool that combines solar and wind forecasts with energy storage optimization. The system predicts optimal charging and discharging cycles for batteries, ensuring consistent power delivery even during cloudy or low-wind periods.
4. Benefits of AI and Predictive Analytics in Solar Energy
The integration of AI and predictive analytics offers numerous advantages:
a. Increased Efficiency
AI optimizes every aspect of solar operations, from panel orientation to energy distribution. This leads to higher energy yields, lower operational costs, and improved profitability.
b. Enhanced Reliability
With precise forecasting and automated controls, AI ensures a steady flow of solar energy, reducing reliance on backup systems and enhancing overall reliability.
c. Cost Savings
Predictive maintenance minimizes unexpected breakdowns and extends equipment lifespans, resulting in substantial cost savings. Additionally, optimized energy trading strategies help maximize financial returns.
d. Environmental Impact
By enabling greater penetration of solar energy into the grid, AI reduces greenhouse gas emissions and supports global decarbonization goals.
5. Challenges and Considerations
While AI holds immense promise, several challenges must be addressed to fully realize its potential in solar energy management:
a. Data Quality and Availability
AI models require large volumes of accurate, high-quality data. Inconsistent or incomplete datasets can compromise predictions and lead to suboptimal outcomes.
b. Cybersecurity Risks
As solar systems become more interconnected, they become vulnerable to cyberattacks. Robust security measures are essential to protect sensitive data and critical infrastructure.
c. High Initial Costs
Implementing AI solutions often requires significant upfront investments in hardware, software, and expertise. Smaller players in the solar market may struggle to afford these technologies without subsidies or partnerships.
d. Regulatory Barriers
Outdated regulations and lack of standardization can hinder the deployment of AI-driven innovations, particularly in areas like grid integration and energy trading.
6. The Road Ahead
The convergence of AI and solar energy represents a paradigm shift in how we manage renewable resources. As computational power continues to grow and AI algorithms become more sophisticated, their impact on the solar industry will only deepen.
Future developments may include:
- Self-Learning Solar Panels: Panels equipped with embedded AI chips that autonomously adapt to changing environmental conditions.
- AI-Powered Microgrids: Autonomous microgrids that seamlessly integrate solar, storage, and other renewables to serve remote or disaster-prone areas.
- Global Collaboration Platforms: AI-driven platforms that aggregate insights from solar installations worldwide, fostering knowledge sharing and innovation.
7. Conclusion: Harnessing the Power of Intelligence
AI and predictive analytics are no longer futuristic concepts—they are here, transforming solar energy management today. By addressing the inherent challenges of solar energy—intermittency, complexity, and scalability—these technologies pave the way for a cleaner, smarter, and more resilient energy ecosystem.
For solar energy specialists, embracing AI is not just an option; it is a necessity. As we strive to meet ambitious climate targets and transition to a carbon-neutral future, the marriage of solar and AI offers a path forward—one illuminated by the limitless potential of human ingenuity and technological progress. The sun provides abundant energy, and with AI as our ally, we are finally poised to harness it to its fullest extent.